1. |
Byra M., Wong J.♦, Shah S.♦, Han A.♦, O Brien W.♦, Du J.♦, Chang E.♦, Andre M.♦, High-frequency quantitative ultrasound and B-mode analysis for characterization of peripheral nerves including carpal tunnel syndrome,
ASA, 178th Meeting of the Acoustical Society of America, 2019-12-02/12-06, San Diego (US), DOI: 10.1121/1.5136729, Vol.146, No.4, pp.2809-2809, 2019 Abstract: We investigated the use of high-frequency quantitative ultrasound (QUS) and B-mode texture features to characterize ulnar and median nerve fascicles using a clinical scanner (Vevo MD) and a 30-MHz center-frequency probe. US correlation with histology was first investigated in the ulnar nerve in situ in cadaveric specimens. 85 fascicles were matched in B-mode images and the histology sections. Collagen and myelin concentrations were quantified from trichrome labeling, and backscatter coefficient (-24.89 ± 8.31 dB), attenuation coefficient (0.92 ± 0.04 dB/cm MHz), Nakagami parameter (1.01 ± 0.18) and entropy (6.92 ± 0.83) were calculated from ultrasound data. B-mode texture features were obtained via the gray-level co-occurrence matrix algorithm. Combined collagen and myelin concentration were significantly correlated with the backscatter coefficient (R = -0.68), entropy (R = -0.51), and several texture features. For the median nerve, we measured backscatter and morphology in 10 patients with carpal tunnel syndrome and 21 healthy volunteers. Significant differences (<0.01) between patients and controls and AUC 0.89–0.94 for QUS biomarkers were observed. Our study indicates that QUS may potentially provide useful information on structural components of even very small nerves (2 × 4 mm) and fascicles for diagnosing and monitoring injury, and surgical planning. Affiliations:
Byra M. | - | IPPT PAN | Wong J. | - | University of California (US) | Shah S. | - | University of California (US) | Han A. | - | University of Illinois at Urbana-Champaign (US) | O Brien W. | - | University of Illinois at Urbana-Champaign (US) | Du J. | - | University of California (US) | Chang E. | - | University of California (US) | Andre M. | - | University of California (US) |
| 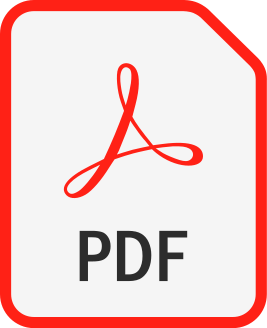 |
2. |
Byra M., Han A.♦, Boehringer A.♦, Zhang Y.♦, Erdman J.♦, Loomba R.♦, Valasek M.♦, Sirlin C.♦, O Brien W.♦, Andre M.♦, Quantitative liver fat fraction measurement by multi-view sonography using deep learning and attention maps,
ASA, 178th Meeting of the Acoustical Society of America, 2019-12-02/12-06, San Diego (US), DOI: 10.1121/1.5136936, Vol.146, No.4, pp.2809-1, 2019 Abstract: Qualitative sonography is used to assess nonalcoholic fatty liver disease (NAFLD), an important health issue worldwide. We used B-mode image deep-learning to objectively assess NAFLD in 4 views of the liver (hepatic veins at confluence with inferior vena cava, right portal vein, right posterior portal vein and liver/kidney) in 135 patients with known or suspected NAFLD. Transfer learning with a deep convolutional neural network (CNN) was applied for quantifying fat fraction and diagnosing fatty liver (≥ 5%) using contemporaneous MRI-PDFF as ground truth. Single and multi-view learning approaches were compared. Class activation mapping generated attention maps to highlight regions important for deep learning-based recognition. The most accurate single view was hepatic veins, with area under the receiver operating characteristic curve (AUC) of 0.86 and Spearman’s rank correlation coefficient of 0.65. A multi-view ensemble of deep-learning models trained for each view separately improved AUC (0.93) and correlation coefficient (0.76). Attention maps highlighted regions known to be used by radiologists in their qualitative assessment, e.g., hepatic vein-parenchyma interface and liver-kidney interface. Machine learning of four liver views can automatically and objectively assess liver fat. Class activation mapping suggests that the CNN focuses on similar features as radiologists. [No. R01DK106419.] Affiliations:
Byra M. | - | IPPT PAN | Han A. | - | University of Illinois at Urbana-Champaign (US) | Boehringer A. | - | University of California (US) | Zhang Y. | - | University of California (US) | Erdman J. | - | University of Illinois at Urbana-Champaign (US) | Loomba R. | - | University of California (US) | Valasek M. | - | University of California (US) | Sirlin C. | - | University of California (US) | O Brien W. | - | University of Illinois at Urbana-Champaign (US) | Andre M. | - | University of California (US) |
| 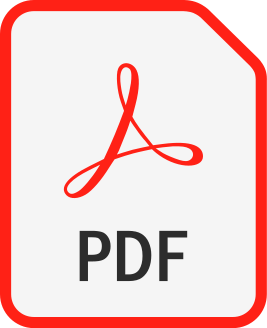 |