1. |
Byra M., Jarosik P., Karwat P., Klimonda Z., Lewandowski M., Implicit Neural Representations for Speed-of-Sound Estimation in Ultrasound,
UFFC-JS, 2024 IEEE Ultrasonics, Ferroelectrics, and Frequency Control Joint Symposium, 2024-09-22/09-26, Tajpej (TW), pp.1-4, 2024 Abstract: Accurate estimation of the speed-of-sound (SoS) is important for ultrasound (US) image reconstruction techniques and tissue characterization. Various approaches have been proposed to calculate SoS, ranging from tomography-inspired algorithms like CUTE to convolutional networks, and more recently, physics-informed optimization frameworks based on differentiable beamforming. In this work, we utilize implicit neural representations (INRs) for SoS estimation in US. INRs are a type of neural network architecture that encodes continuous functions, such as images or physical quantities, through the weights of a network. Implicit networks may overcome the current limitations of SoS estimation techniques, which mainly arise from the use of non-adaptable and oversimplified physical models of tissue. Moreover, convolutional networks for SoS estimation, usually trained using simulated data, often fail when applied to real tissues due to out-of-distribution and data-shift issues. In contrast, implicit networks do not require extensive training datasets since each implicit network is optimized for an individual data case. This adaptability makes them suitable for processing US data collected from varied tissues and across different imaging protocols. We evaluated the proposed SoS estimation method based on INRs using data collected from a tissue-mimicking phantom containing four cylindrical inclusions, with SoS values ranging from 1480 m/s to 1600 m/s. The inclusions were immersed in a material with an SoS value of 1540 m/s. In experiments, the proposed method achieved strong performance, clearly demonstrating the usefulness of implicit networks for quantitative US applications. Keywords: beamforming, deep learning, implicit neural representations, speed-of-sound, quantitative ultrasound Affiliations:
Byra M. | - | IPPT PAN | Jarosik P. | - | IPPT PAN | Karwat P. | - | IPPT PAN | Klimonda Z. | - | IPPT PAN | Lewandowski M. | - | IPPT PAN |
| 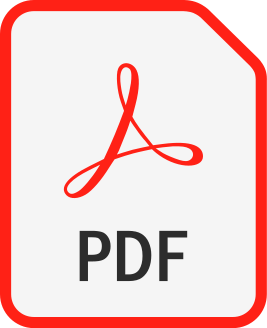 |
2. |
Jarosik P., Byra M., Klimonda Z., Dłużewski P., Lewandowski M., Deep Reinforcement Learning Approach for Adaptive Ultrasound Image Reconstruction with a Flexible Array Probe,
UFFC-JS, 2024 IEEE Ultrasonics, Ferroelectrics, and Frequency Control Joint Symposium, 2024-09-22/09-26, Tajpej (TW), No.8573, pp.62-62, 2024 Abstract: Background, Motivation and Objective
Flexible ultrasound (US) arrays are a promising technology that may further democratize US technology — e.g. in wearable US. Flexible transducers also pose challenges in image reconstruction, as they require adaptable beamforming delays due to a changing geometry of the probe. Various approaches have been proposed for flexible array shape estimation and beamforming, e.g. external sensors, deep learning and optimization. In this work, we propose a deep reinforcement learning (DRL) approach, where a software agent is responsible for tracking the array shape to properly reconstruct US B-mode image.
Statement of Contribution/Methods
Here we considered a reinforcement learning environment as a setup consisting of a US system with the flexible array and point targets phantom. The environment was simulated using j-Wave software. The environment’s state consisted of the current shape of the array, modeled as a sinusoid s = a sin(bx + c), and the current model of the array assumed by the beamformer: s’ = a’ sin(b’x + c’) (single-element STA scheme). A single episode consisted of
7 steps; the parameters a, b, c could vary from step to step (within bounds of physical constraints). The agent observed the current B-mode, could modify the current values of a’, b’ and c’ (action) and received a reward equal to the linear combination of the coherence factor and structural similarity index measure (SSIM) between the current and reference image. We trained our agent using TD3 approach and tested it for various settings of a, b and c.
Results/Discussion
Our agent achieved an average SSIM of 0.73 per episode step. Figure 1 shows the sequence of states and images within an example episode; the agent was able to correctly react to the change of the array shape. The DRL approach has the following advantages compared to other methods: the agent can be trained to operate in an environment with a changing state; and the agent can be trained to maximize expected return (dependent on beamforming quality metric), which does not have to be differentiable.
Fig. 1. (Top) B-mode images, estimated array shape (red) and actual shape (black) within an example episode. (Bottom) Coherence factor and SSIM achieved by the agent during the episode Affiliations:
Jarosik P. | - | IPPT PAN | Byra M. | - | IPPT PAN | Klimonda Z. | - | IPPT PAN | Dłużewski P. | - | IPPT PAN | Lewandowski M. | - | IPPT PAN |
| 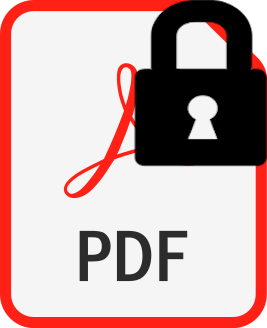 |
3. |
Jarosik P., Lewandowski M., Klimonda Z., Byra M., Pixel-wise deep reinforcement learning approach for ultrasound image denoising,
IUS, IEEE International Ultrasonics Symposium (IUS), 2021, 2021-09-11/09-16, on-line (US), DOI: 10.1109/IUS52206.2021.9593591, pp.1-4, 2021 Abstract: Ultrasound (US) imaging is widely used for the tissue characterization. However, US images commonly suffer from speckle noise, which degrades perceived image quality. Various deep learning approaches have been proposed for US image denoising, but most of them lack the interpretability of how the network is processing the US image (black box problem). In this work, we utilize a deep reinforcement learning (RL) approach, the pixelRL, to US image denoising. The technique utilizes a set of easily interpretable and commonly used filtering operations applied in a pixel-wise manner. In RL, software agents act in an unknown environment and receive appropriate numerical rewards. In our case, each pixel of the input US image has an agent and state of the environment is the current US image. Agents iteratively denoise the US image by executing the following pixel-wise pre-defined actions: Gaussian, bilateral, median and box filtering, pixel value increment/decrement and no action. The proposed approach can be used to generate action maps depicting operations applied to process different parts of the US image. Agents were pre-trained on natural gray-scale images and evaluated on the breast mass US images. To enable the evaluation, we artificially corrupted the US images with noise. Compared with the reference (noise free US images), filtration of the images with the proposed method increased the average peak signal-to-noise ratio (PSNR) score from 14 dB to 26 dB and increased the structure similarity index score from 0.22 to 0.54. Our work confirms that it is feasible to use pixel-wise RL techniques for the US image denoising. Keywords: deep reinforcement learning, ultrasound imaging, image denoising, filtration, breast cancer Affiliations:
Jarosik P. | - | IPPT PAN | Lewandowski M. | - | IPPT PAN | Klimonda Z. | - | IPPT PAN | Byra M. | - | IPPT PAN |
| 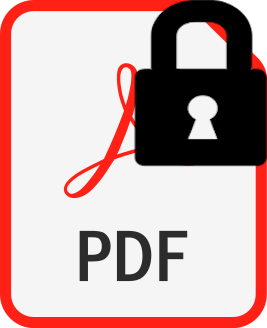 |
4. |
Lewandowski M., Jarosik P.♦, Tasinkevych Y., Walczak M., Efficient GPU implementation of 3D spectral domain synthetic aperture imaging,
IUS 2020, IEEE International Ultrasonics Symposium, 2020-09-07/09-11, Las Vegas (US), DOI: 10.1109/IUS46767.2020.9251552, pp.1-3, 2020 Abstract: In this work, we considered the implementation of a 3D volume reconstruction algorithm for single plane-wave ultrasound insonification. We review the theory behind the Hybrid Spectral-Domain Imaging (HSDI) algorithm, provide details of the algorithm implementation for Nvidia CUDA GPU cards, and discuss the performance evaluation results. The average time required to reconstruct a single data volume using our GPU implementation of the HSDI algorithm was 22 ms. We also present an iso-surface extraction result using a marching cubes algorithm. Our work constitutes a preliminary research for further development and implementation of 3D volume reconstruction using GPU implementation of the spectral domain imaging algorithm. Keywords: ultrasound imaging, 3D ultrasound, volumetric imaging, gpu Affiliations:
Lewandowski M. | - | IPPT PAN | Jarosik P. | - | other affiliation | Tasinkevych Y. | - | IPPT PAN | Walczak M. | - | IPPT PAN |
| 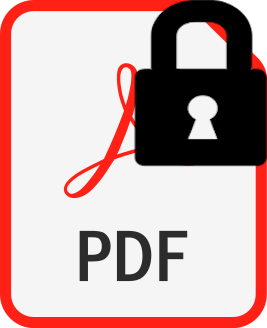 |
5. |
Jarosik P.♦, Lewandowski M., Automatic Ultrasound Guidance Based on Deep Reinforcement Learning,
IUS 2019, IEEE, International Ultrasonics Symposium, 2019-10-06/10-09, Glasgow (GB), DOI: 10.1109/ULTSYM.2019.8926041, pp.475-478, 2019 Abstract: Ultrasound is becoming the modality of choice for everyday medical diagnosis, due to its mobility and decreasing price. As the availability of ultrasound diagnostic devices for untrained users grows, appropriate guidance becomes desirable. This kind of support could be provided by a software agent, who easily adapts to new conditions, and whose role is to instruct the user on how to obtain optimal settings of the imaging system during an examination. In this work, we verified the feasibility of implementing and training such an agent for ultrasound, taking the deep reinforcement learning approach. The tasks it was given were to find the optimal position of the transducer’s focal point (FP task) and to find an appropriate scanning plane (PP task). The ultrasound environment consisted of a linear-array transducer acquiring information from a tissue phantom with cysts forming an object-of-interest (OOI). The environment was simulated in the Field-II software. The agent could perform the following actions: move the position of the probe to the left/right, move focal depth upwards/downwards, rotate the probe clockwise/counter-clockwise, or do not move. Additional noise was applied to the current probe setting. The only observations the agent received were B-mode frames. The agent acted according to stochastic policy modeled by a deep convolutional neural network, and was trained using the vanilla policy gradient update algorithm. After the training, the agent’s ability to accurately locate the position of the focal depth and scanning plane improved. Our preliminary results confirmed that deep reinforcement learning can be applied to the ultrasound environment. Keywords: ultrasound guidance, reinforcement learning, deep learning Affiliations:
Jarosik P. | - | other affiliation | Lewandowski M. | - | IPPT PAN |
| 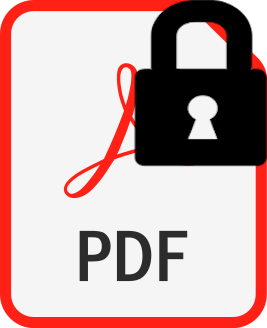 |
6. |
Jarosik P.♦, Lewandowski M., The feasibility of deep learning algorithms integration on a GPU-based ultrasound research scanner,
IUS 2017, IEEE International Ultrasonics Symposium, 2017-09-06/09-09, Washington (US), DOI: 10.1109/ULTSYM.2017.8091750, pp.1-4, 2017 Abstract: Ultrasound medical diagnostics is a real-time modality based on a doctor's interpretation of images. So far, automated Computer-Aided Diagnostic tools were not widely applied to ultrasound imaging. The emerging methods in Artificial Intelligence, namely deep learning, gave rise to new applications in medical imaging modalities. The work's objective was to show the feasibility of implementing deep learning algorithms directly on a research scanner with GPU software beamforming. We have implemented and evaluated two deep neural network architectures as part of the signal processing pipeline on the ultrasound research platform USPlatform (us4us Ltd., Poland). The USPlatform is equipped with a GPU cluster, enabling full software-based channel data processing as well as the integration of open source Deep Learning frameworks. The first neural model (S-4-2) is a classical convolutional network for one-class classification of baby body parts. We propose a simple 6-layer network for this task. The model was trained and evaluated on a dataset consisting of 786 ultrasound images of a fetal training phantom. The second model (Gu-net) is a fully convolutional neural network for brachial plexus localisation. The model uses 'U-net'-like architecture to compute the overall probability of target detection and the probability mask of possible target locations. The model was trained and evaluated on 5640 ultrasound B-mode frames. Both training and inference were performed on a multi-GPU (Nvidia Titan X) cluster integrated with the platform. As performance metrics we used: accuracy as a percentage of correct answers in classification, dice coefficient for object detection, and mean and std. dev. of a model's response time. The 'S-4-2' model achieved 96% classification accuracy and a response time of 3 ms (334 predictions/s). This simple model makes accurate predictions in a short time. The 'Gu-net' model achieved a 0.64 dice coefficient for object detection and a 76% target's presence classification accuracy with a response time of 15 ms (65 predictions/s). The brachial plexus detection task is more challenging and requires more effort to find the right solution. The results show that deep learning methods can be successfully applied to ultrasound image analysis and integrated on a single advanced research platform Keywords: Ultrasonic imaging, Neural networks, Convolution, Machine learning, Image segmentation, Kernel Affiliations:
Jarosik P. | - | other affiliation | Lewandowski M. | - | IPPT PAN |
| 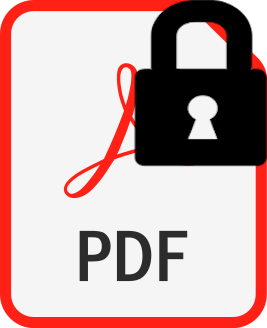 |