1. |
Deshpande S.♦, Rappel H.♦, Hobbs M.♦, Bordas S.♦, Lengiewicz J.A., Gaussian process regression + deep neural network autoencoder for probabilistic surrogate modeling in nonlinear mechanics of solids,
COMPUTER METHODS IN APPLIED MECHANICS AND ENGINEERING, ISSN: 0045-7825, DOI: 10.1016/j.cma.2025.117790, Vol.437, No.117790, pp.1-17, 2025 Streszczenie: Many real-world applications demand accurate and fast predictions, as well as reliable uncertainty estimates. However, quantifying uncertainty on high-dimensional predictions is still a severely under-investigated problem, especially when input–output relationships are non-linear. To handle this problem, the present work introduces an innovative approach that combines autoencoder deep neural networks with the probabilistic regression capabilities of Gaussian processes. The autoencoder provides a low-dimensional representation of the solution space, while the Gaussian process is a Bayesian method that provides a probabilistic mapping between the low-dimensional inputs and outputs. We validate the proposed framework for its application to surrogate modeling of non-linear finite element simulations. Our findings highlight that the proposed framework is computationally efficient as well as accurate in predicting non-linear deformations of solid bodies subjected to external forces, all the while providing insightful uncertainty assessments. Słowa kluczowe: Surrogate modeling,Deep neural networks,Gaussian proces,Autoencoders,Uncertainty quantification,Finite element method Afiliacje autorów:
Deshpande S. | - | University of Luxembourg (LU) | Rappel H. | - | inna afiliacja | Hobbs M. | - | inna afiliacja | Bordas S. | - | inna afiliacja | Lengiewicz J.A. | - | IPPT PAN |
| 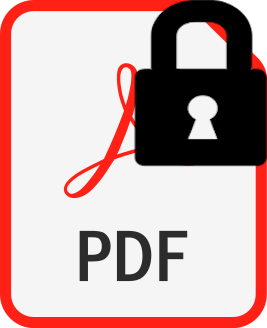 | 200p. |
2. |
Deshpande S.♦, Bordas S.♦, Lengiewicz J. A., MAgNET: A graph U-Net architecture for mesh-based simulations,
Engineering Applications of Artificial Intelligence, ISSN: 0952-1976, DOI: 10.1016/j.engappai.2024.108055, Vol.133 B, No.108055, pp.1-18, 2024 Streszczenie: In many cutting-edge applications, high-fidelity computational models prove to be too slow for practical use and are therefore replaced by much faster surrogate models. Recently, deep learning techniques have increasingly been utilized to accelerate such predictions. To enable learning on large-dimensional and complex data, specific neural network architectures have been developed, including convolutional and graph neural networks. In this work, we present a novel encoder–decoder geometric deep learning framework called MAgNET, which extends the well-known convolutional neural networks to accommodate arbitrary graph-structured data. MAgNET consists of innovative Multichannel Aggregation (MAg) layers and graph pooling/unpooling layers, forming a graph U-Net architecture that is analogous to convolutional U-Nets. We demonstrate the predictive capabilities of MAgNET in surrogate modeling for non-linear finite element simulations in the mechanics of solids. Słowa kluczowe: Geometric deep learning, Mesh based simulations, Finite element method, Graph U-Net, Surrogate modeling Afiliacje autorów:
Deshpande S. | - | University of Luxembourg (LU) | Bordas S. | - | inna afiliacja | Lengiewicz J. A. | - | IPPT PAN |
| 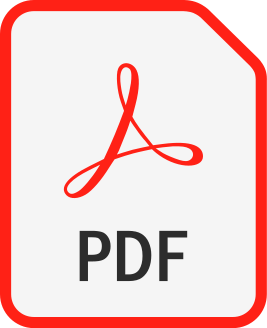 | 140p. |
3. |
Deshpande S.♦, Sosa R.♦, Bordas S.P.♦, Lengiewicz J.A., Convolution, aggregation and attention based deep neural networks for accelerating simulations in mechanics,
Frontiers in Materials, ISSN: 2296-8016, DOI: 10.3389/fmats.2023.1128954, Vol.10, No.1128954, pp.1-12, 2023 Streszczenie: Deep learning surrogate models are being increasingly used in accelerating scientific simulations as a replacement for costly conventional numerical techniques. However, their use remains a significant challenge when dealing with real-world complex examples. In this work, we demonstrate three types of neural network architectures for efficient learning of highly non-linear deformations of solid bodies. The first two architectures are based on the recently proposed CNN U-NET and MAgNET (graph U-NET) frameworks which have shown promising performance for learning on mesh-based data. The third architecture is Perceiver IO, a very recent architecture that belongs to the family of attention-based neural networks–a class that has revolutionised diverse engineering fields and is still unexplored in computational mechanics. We study and compare the performance of all three networks on two benchmark examples, and show their capabilities to accurately predict the non-linear mechanical responses of soft bodies. Słowa kluczowe: surrogate modeling, deep learning-artificial neural network, CNN U-NET, graph U-net, perceiver IO, finite element method Afiliacje autorów:
Deshpande S. | - | University of Luxembourg (LU) | Sosa R. | - | inna afiliacja | Bordas S.P. | - | University of Luxembourg (LU) | Lengiewicz J.A. | - | IPPT PAN |
| 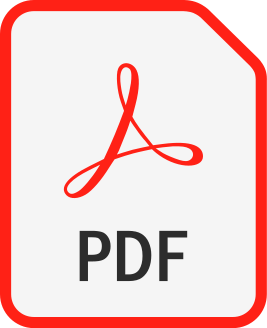 | 70p. |
4. |
Deshpande S.♦, Lengiewicz J., Bordas S.P.A.♦, Probabilistic deep learning for real-time large deformation simulations,
COMPUTER METHODS IN APPLIED MECHANICS AND ENGINEERING, ISSN: 0045-7825, DOI: 10.1016/j.cma.2022.115307, Vol.398, pp.115307-1-115307-26, 2022 Streszczenie: For many novel applications, such as patient-specific computer-aided surgery, conventional solution techniques of the underlying nonlinear problems are usually computationally too expensive and are lacking information about how certain can we be about their predictions. In the present work, we propose a highly efficient deep-learning surrogate framework that is able to accurately predict the response of bodies undergoing large deformations in real-time. The surrogate model has a convolutional neural network architecture, called U-Net, which is trained with force–displacement data obtained with the finite element method. We propose deterministic and probabilistic versions of the framework. The probabilistic framework utilizes the Variational Bayes Inference approach and is able to capture all the uncertainties present in the data as well as in the deep-learning model. Based on several benchmark examples, we show the predictive capabilities of the framework and discuss its possible limitations. Słowa kluczowe: convolutional neural network, Bayesian inference, Bayesian deep learning, large deformations, finite element method, real-time simulations Afiliacje autorów:
Deshpande S. | - | University of Luxembourg (LU) | Lengiewicz J. | - | IPPT PAN | Bordas S.P.A. | - | University of Luxembourg (LU) |
| 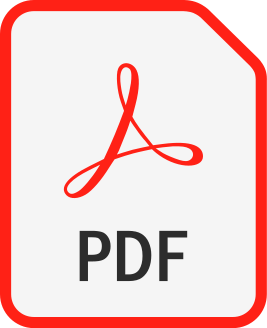 | 200p. |